Advanced process control - deep learning algorithms for large scale processes: A refinery case study
Dec
8
2021
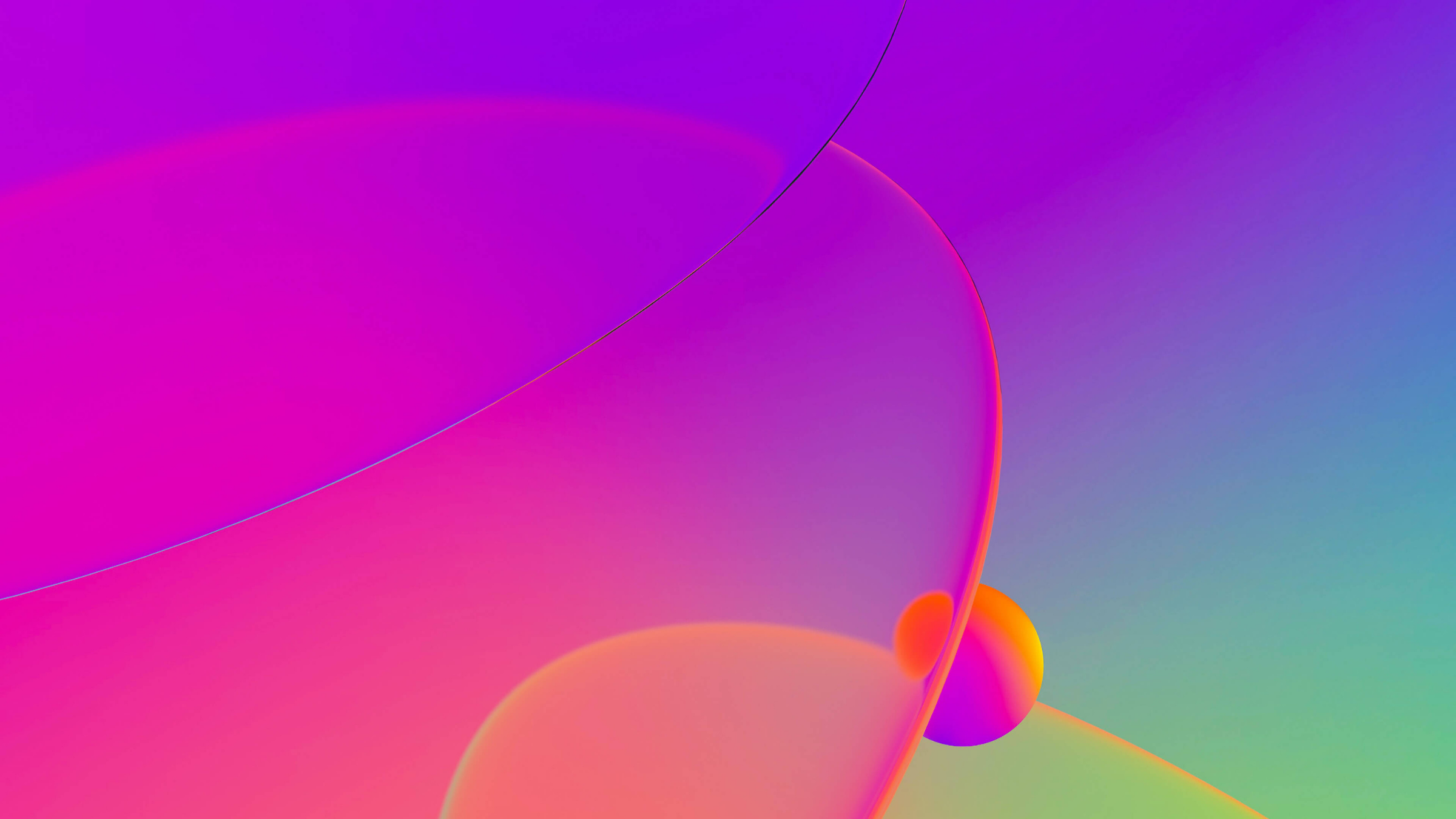
Dec
8
2021
Description
Stringent environmental regulations, economic uncertainty, market competition, raw materials disruptions etc. have forced chemical companies to enhance the efficiency of its operation in order to maintain profits. One way to do so is to improve production planning and process control. Historically, industrial advanced process control has been implemented using linear models of the process/plant. Linear models provide several advantages (e.g. interpretability, fast optimization times); however, they are not able to capture the nonlinear nature of large-scale industrial processes. This is a key feature since frequently the nonlinear behavior of the plant actually captures the most relevant (in terms of efficiency and economics) operating regimes. Hence, the implementation of nonlinear models is necessary to improve the efficiency of the operation. Rigorous first principles models can be developed and implemented for advanced process control; nevertheless, the computational complexity of the resulting framework prohibits its real industrial application. A means to bypass the computational challenge is to use machine-learning models, in particular, deep learning models derived from process data. In the present study, we propose two advanced process control algorithms that integrate deep learning models. One of the algorithms implements only deep learning models whereas the other one implements deep learning and linear models (i.e., a hybrid algorithm). The algorithms are applied to a large-scale industrial process (the production of fuels such as gasoline and diesel). Several scenarios are simulated (with different process conditions) for each one of the algorithms using a first-principles model as the “plant.” In addition, the deep learning algorithms are benchmarked against the currently implemented linear advanced process control algorithm. The results demonstrate that the deep learning algorithms outperform (in terms of revenue) by at least 0.3% the linear algorithm for any scenario (which results in a substantial economic improvement for the process operation). Finally, the deep learning algorithms also show promising solution times which are necessary for real process implementation.
Other Events in This Series
Dec
5
2023
Fall Graduate Portfolio Colloquium - Shreya Shukla
System Evaluation by Betting Mechanism Portfolio in Scientific Computation
11:30 am – 12:00 pm • Virtual
Speaker(s): Shreya Shukla
Dec
5
2023
Fall Graduate Portfolio Colloquium - Damla Cinoglu
System Evaluation by Betting Mechanism Portfolio in Scientific Computation
12:00 pm – 12:30 pm • Virtual
Speaker(s): Damla Cinoglu
Dec
5
2023
Fall Graduate Portfolio Colloquium - Zexi Zhou
Daily Worry, Rumination, and Sleep in Late Life
12:30 pm – 1:00 pm • Virtual
Speaker(s): Zexi Zhou
Dec
5
2023
Fall Graduate Portfolio Colloquium - Natasha Nhu Bui
Binge Drinking and Preventive Cancer Screenings among Women in the United States by Race and Ethnicity
1:00 pm – 1:30 pm • Virtual
Speaker(s): Natasha Nhu Bui