Statistics Ph.D. Dissertation Defense - Xizewen Han
Nov
18
2024
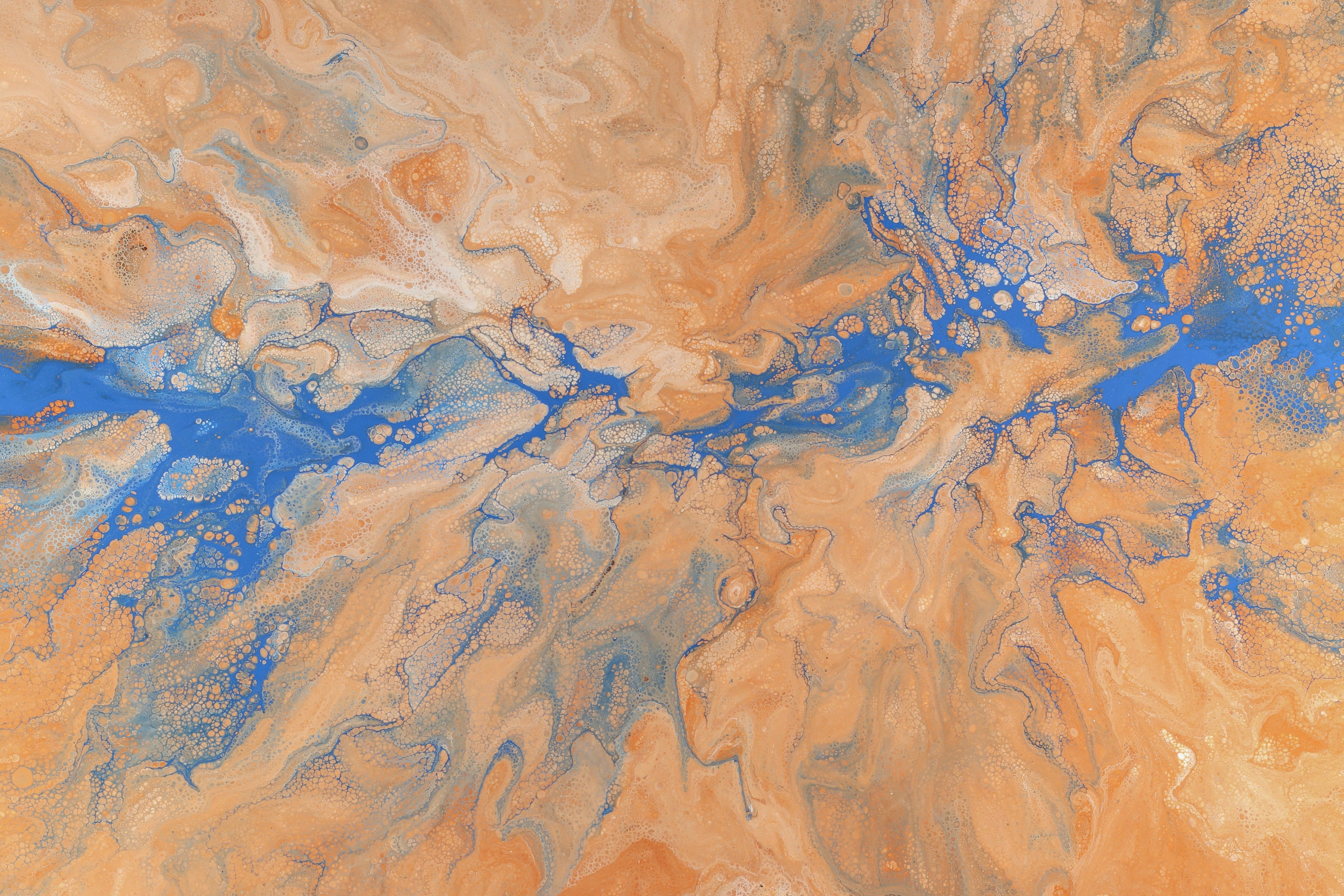
Nov
18
2024
Description
This 2024 Dissertation Defense will be held on Monday November 18, from 11:00 a.m. to 1:00 p.m. with Xizewen Han. This event will be hybrid. If you are able to attend in person, it will be held in WEL 5.258. If you need the Zoom link, please email stat.admin@austin.utexas.edu.
Title: Classification and Regression Diffusion Models and Tree-Based Extensions
Advisor: Dr. Mingyuan Zhou
Abstract: In supervised learning, assuming an explicit parametric form for the target conditional distribution p(y|x) has been a standard approach. In practice, such distributional assumptions can sometimes be overly restrictive.
This dissertation introduces a new paradigm for supervised learning through a diffusion-based generative modeling framework, free from parametric assumptions on p(y|x). This framework methodically decomposes the data generation process into manageable and structured incremental steps via a Bayesian formulation, with each step assuming a Gaussian distribution that serves as an atomic and fundamental building block. This framework is applicable to both regression and classification.
Under this paradigm, two classes of generative models for supervised learning are presented: Classification and Regression Diffusion (CARD) models and Diffusion Boosted Trees (DBT), parameterized by deep neural networks and decision trees, respectively. These models demonstrate strong performance in learning distributions with diverse statistical characteristics in regression and offer instance-level confidence for decision making in classification. Additionally, a toolkit for evaluating model performance based solely on data samples is proposed, providing practical methods for assessing the effectiveness of these models.
Location
This event will be hybrid. If you are able to attend in person, it will be held in WEL 5.258. If you need the Zoom link, please email stat.admin@austin.utexas.edu.