Latent Variable Modeling with Random Features
Sep
30
2022
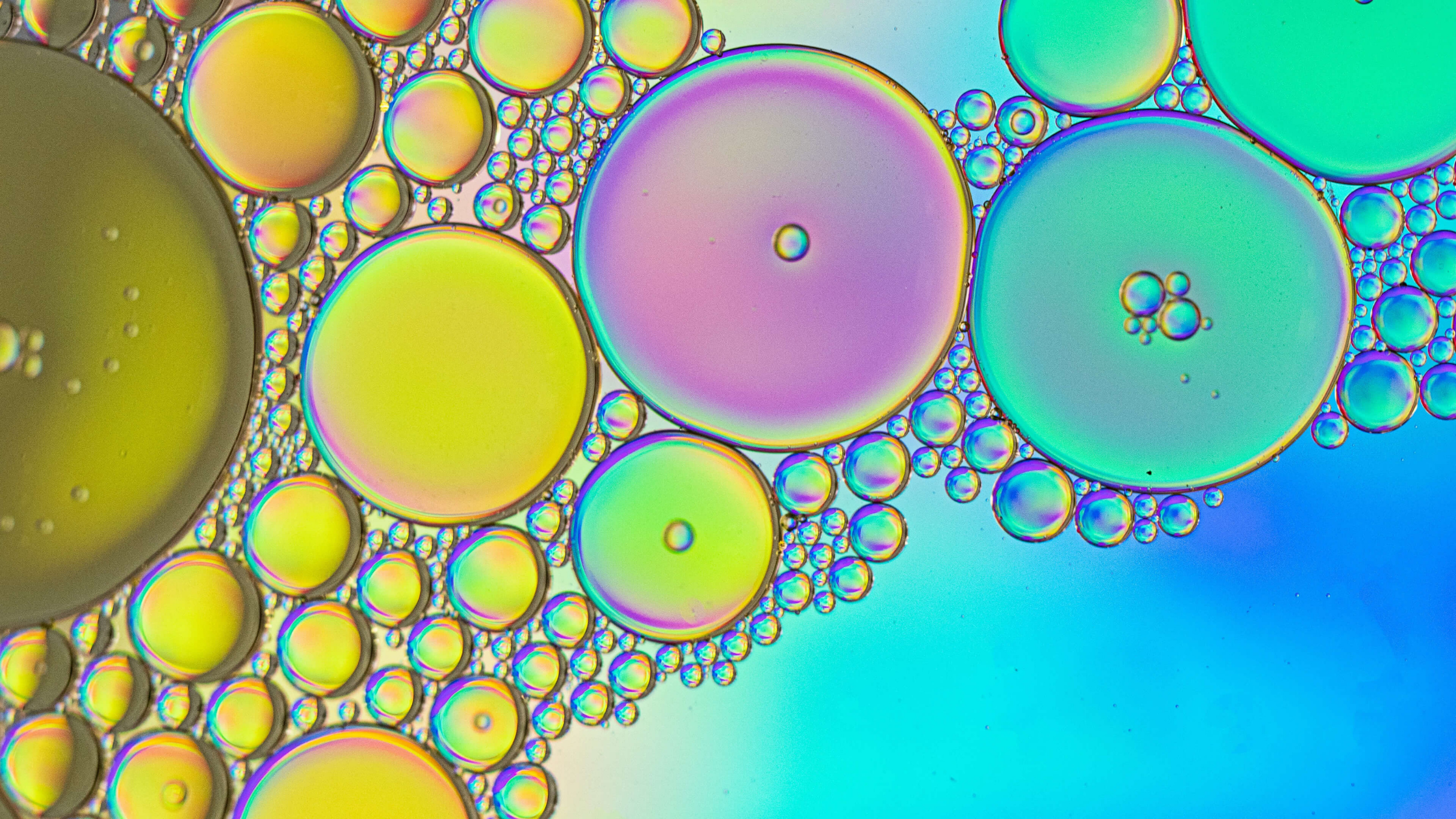
Sep
30
2022
Description
The Fall 2022 SDS Seminar Series continues on Friday, September 30th from 2:00 p.m. to 3:00 p.m. with Dr. Michael Zhang (Assistant Professor in the Department of Statistics and Actuarial Science at the University of Hong Kong). This event is in-person, but a virtual option will be available as well.
Title: Latent variable modeling with random features
Abstract: Gaussian process-based latent variable models are flexible and theoretically grounded tools for nonlinear dimension reduction, but generalizing to non-Gaussian data likelihoods within this nonlinear framework is statistically challenging. Here, we use random features to develop a family of nonlinear dimension reduction models that are easily extensible to non-Gaussian data likelihoods; we call these random feature latent variable models (RFLVMs). By approximating a nonlinear relationship between the latent space and the observations with a function that is linear with respect to random features, we induce closed-form gradients of the posterior distribution with respect to the latent variable. This allows the RFLVM framework to support computationally tractable nonlinear latent variable models for a variety of data likelihoods in the exponential family without specialized derivations. Our generalized RFLVMs produce results comparable with other state-of-the-art dimension reduction methods on diverse types of data, including neural spike train recordings, images, and text data.
Location
Please contact stat.admin@austin.utexas.edu for the seminar link.
Avaya Auditorium (POB 2.302)
Share
Other Events in This Series
No events to display