Seminar Series - Dr. Connor Jerzak
Mar
3
2023
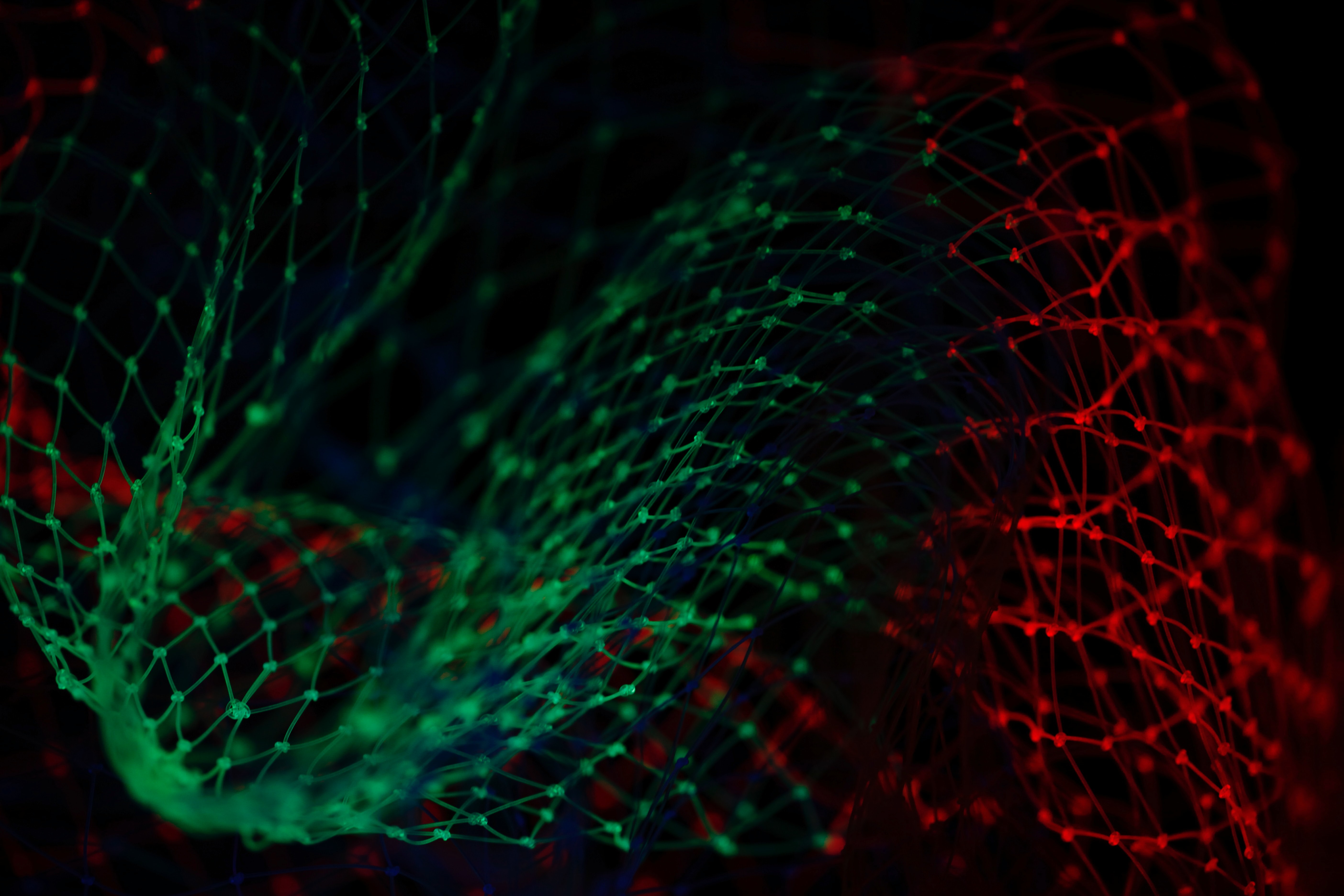
Photo by Pietro Jeng on Unsplash
Mar
3
2023
Description
The Spring 2023 SDS Seminar Series continues on Friday, March 3rd from 2:00 p.m. to 3:00 p.m. with Dr. Connor Jerzak (Assistant Professor at University of Texas at Austin). This event is in-person, but a virtual option will be available as well.
Title: Optimal Stochastic Interventions with High-Dimensional Factorial Experiments: Application to Conjoint Analysis
Abstract: Although there exists a rapidly growing literature on policy learning, much of the existing work focuses on the determination of an optimal treatment rule that determines who should be treated based on their individual characteristics. Instead, this paper studies the problem of finding an optimal intervention regime in high-dimensional factorial experiments. Our motivating application is conjoint analysis, which is popular in marketing and social science research. In one such experiment, respondents were asked to choose between hypothetical political candidates with randomly selected attributes, which include partisanship, policy positions, as well as candidate gender and race. Because the number of unique treatment combinations exceeds the total number of observations, it is impossible to identify the optimal treatment combination in this setting. Instead, we seek to find an optimal stochastic intervention, representing a probability distribution of treatments, that yields the greatest expected utility given variance constraints. We consider two classes of stochastic interventions. In the first, the optimal policy selection is performed in an average case sense---if respondents choose between one of two entities in a forced choice conjoint, we maximize the utility of a reference candidate averaging over features of the opponent. In the second, the optimal policy selection is performed in an adversarial manner in two rounds of selection---we maximize the support of a reference candidate against an opponent group which is also maximizing the support of their candidate in the same target population. We discuss several point estimate and variance-covariance estimators, some of which are obtained in closed form. Finite sample performance is explored via simulation. Our empirical analysis shows the usefulness of the proposed methodologies in the context of the US vote choice for president.