SDS Seminar Series – Po-Ling Loh, University of Cambridge
Mar
28
2025
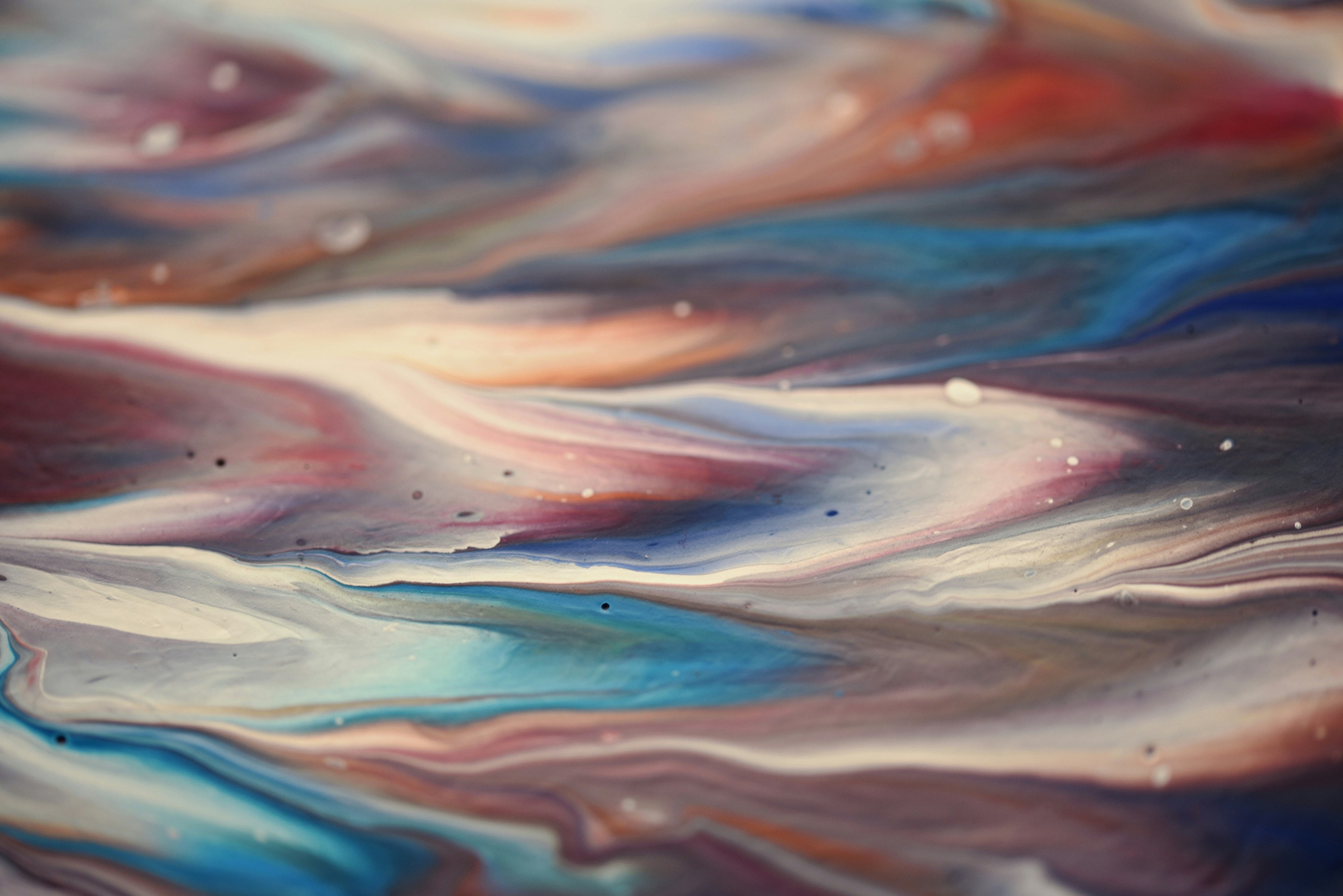
Mar
28
2025
Description
The Spring 2025 SDS Seminar Series continues on March 28th from 2:00 p.m. to 3:00 p.m. with Dr. Poh-Ling Loh (Professor, Department of Pure Mathematics and Mathematical Statistics, University of Cambridge). This event is in-person in the Avaya Room (POB 2.302).
Title: Differentially Private M-estimation via Noisy Optimization
Abstract: We present a noisy composite gradient descent algorithm for differentially private statistical estimation in high dimensions. We begin by providing general rates of convergence for the parameter error of successive iterates under assumptions of local restricted strong convexity and local restricted smoothness. Our analysis is local, in that it ensures a linear rate of convergence when the initial iterate lies within a constant-radius region of the true parameter. At each iterate, multivariate Gaussian noise is added to the gradient in order to guarantee that the output satisfies Gaussian differential privacy. We then derive consequences of our theory for linear regression and mean estimation. Motivated by M-estimators used in robust statistics, we study loss functions which downweight the contribution of individual data points in such a way that the sensitivity of function gradients is guaranteed to be bounded, even without the usual assumption that our data lie in a bounded domain. We prove that the objective functions thus obtained indeed satisfy the restricted convexity and restricted smoothness conditions required for our general theory. We will also discuss the benefits of acceleration in optimization procedures, specifically a private version of the Frank-Wolfe algorithm, and its consequences for statistical estimation.
This is based on joint work with Marco Avella-Medina, Casey Bradshaw, Zheng Liu, and Laurentiu Marchis.
Other Events in This Series
Sep
8
2023
SDS Seminar Series – Dr. Emily Roberts
A Causal Inference Approach for Surrogate Marker Evaluation with Mixed Models
2:00 pm – 3:00 pm • In Person
Speaker(s): Emily Roberts
Sep
15
2023
SDS Seminar Series – Dr. Dimitris Korobilis
Monitoring Multicountry Macroeconomic Risk
2:00 pm – 3:00 pm • Virtual
Speaker(s): Dimitris Korobilis
Sep
22
2023
SDS Seminar Series – Dr. Will Fithian
Estimating the False Discovery Rate of Model Selection
2:00 pm – 3:00 pm • In Person
Speaker(s): Will Fithian
Sep
29
2023
SDS Seminar Series – Dr. David Moriarty
A Data Science Journey in Business
2:00 pm – 3:00 pm • In Person
Speaker(s): David Moriarty
Oct
6
2023
SDS Seminar Series – Dr. Amanda Ellis
Navigating the Future of Statistics Education: Leveraging ChatGPT's Advantages and Overcoming Challenges
2:00 pm – 3:00 pm • Virtual
Speaker(s): Amanda Ellis
Oct
20
2023
SDS Seminar Series – Dr. Amy Zhang
Bisimulation and Reinforcement Learning
2:00 pm – 3:00 pm • Virtual
Speaker(s): Amy Zhang
Oct
27
2023
SDS Seminar Series – Dr. Marcelo Medeiros
Global Inflation Forecasting: Benefits from Machine Learning Methods
2:00 pm – 3:00 pm • Virtual
Speaker(s): Marcelo Medeiros
Nov
3
2023
SDS Seminar Series - Dr. Steve Yadlowsky
Choosing a Proxy Metric from Past Experiments
2:00 pm – 3:00 pm • Virtual
Speaker(s): Steve Yadlowsky
Nov
10
2023
SDS Seminar Series – Drew Herren
Statistical Aspects of SHAP: Functional ANOVA for Model Interpretation
2:00 pm – 3:00 pm • In Person
Speaker(s): Drew Herren
Dec
1
2023
SDS Seminar Series – Dr. Dave Zhao
High-Dimensional Nonparametric Empirical Bayes Problems in Genomics
2:00 pm – 3:00 pm • In Person
Speaker(s): Dave Zhao