Statistics Ph.D. Dissertation Defense - Fuheng Cui
Apr
11
2025
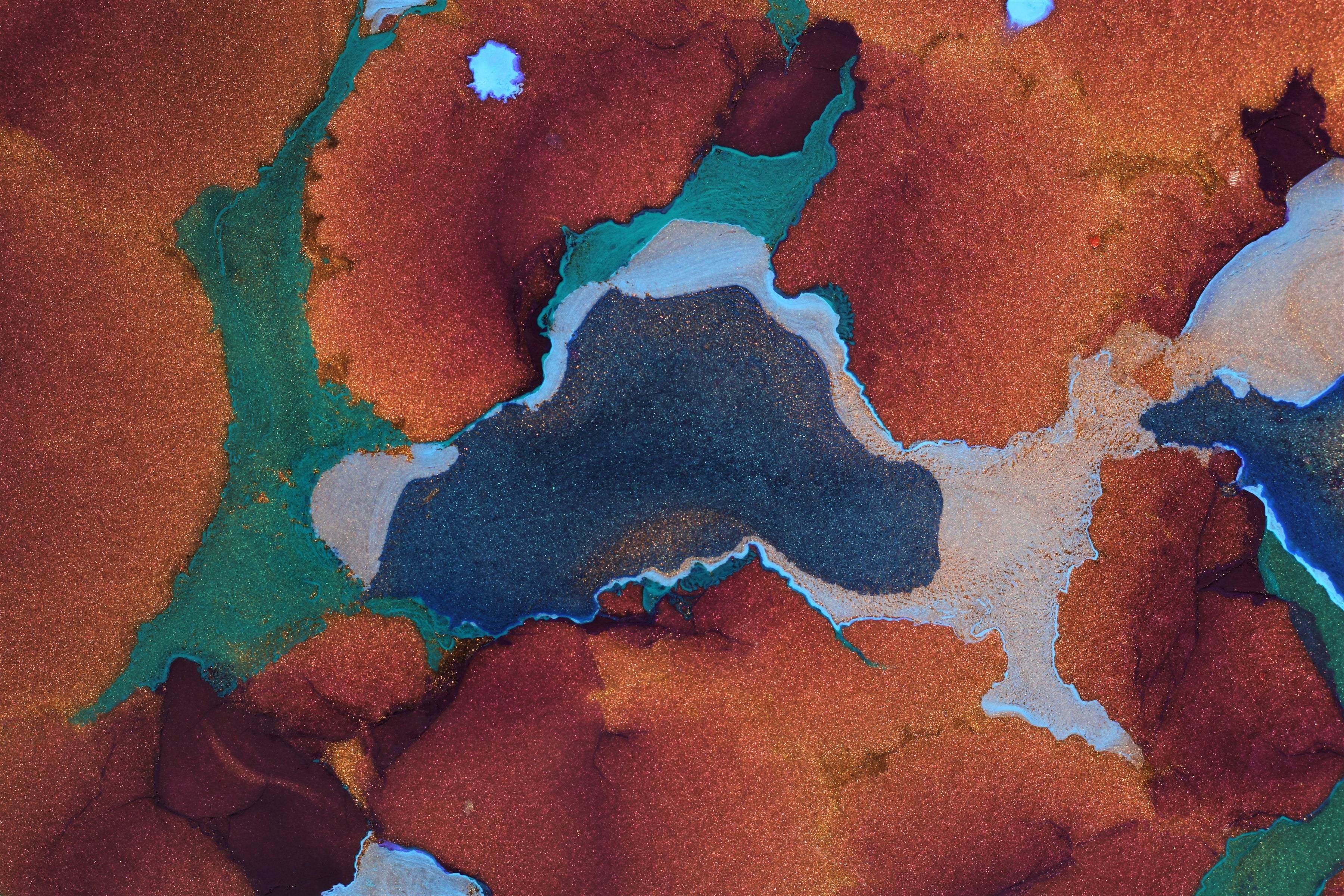
Apr
11
2025
Description
This 2025 Dissertation Defense will be held on Friday, April 11 from 1:00 p.m. to 3:00 p.m. with Fuheng Cui. This event will be hybrid. If you are able to attend in person, it will be held in GDC 7.514 (Bayes Room). If you need the Zoom link, please email stat.admin@austin.utexas.edu.
Title: Bayesian Predictive Posterior Distributions
Advisor: Dr. Stephen Walker
Abstract: Bayesian uncertainty can be characterized in a number of ways, the usual one starting with a prior distribution which represents prior uncertainty as to the value of a parameter. This gets updated to the posterior quantification of uncertainty via the data evidence using the likelihood function. This framework is however difficult to relax. An alternative representation of Bayesian uncertainty has been provided by Doob in 1949 who showed that a predictive sampling scheme also provided a representation of the posterior. This set-up can be relaxed by modifying the nature of a predictive density function. In short, it can be a density estimator given the current knowledge. The idea then is to impute the missing data from the observed sample onwards updating the density estimator as it goes. By modeling the unseen data through this scheme, the object of interest can be computed by the observed data and the simulated unseen data. Under some conditions, we can prove that this procedure is equivalent to sampling from a posterior. In this dissertation, several methods using the predictive sampling scheme are discussed. We first introduce some prerequisite knowledge, such as Bayesian bootstrap, Dirichlet process, discrete-time martingale and its convergence, weak convergence of random measures and exchangeability. Then making use of the advantage of convergence of martingales, the martingale posterior is discussed in the dissertation. Inspired by martingale posteriors, a Bayesian bootstrap for mixture models is introduced as an extension of the traditional Bayesian bootstrap to mixture models. Using submartingales, a new approach to quantify the uncertainty for the log-concave densities is proposed, by which we can directly sample densities from the posterior. A natural martingale posterior constructed by the score function is discussed for Bayesian parametric models as well. In theory, instead of requiring exchangeability in the traditional prior-likelihood-posterior scheme, we only need some weaker conditions such as asymptotic exchangeability in the predictive sampling scheme. In application, these methods can be implemented in parallel and avoid using Markov chain Monte Carlo methods. We prove the convergence and exchangeability for each method. We also provide illustrations and comparisons with the existing methods on both simulated and real data.
Location
This event will be hybrid. If you are able to attend in person, it will be held in GDC 7.514 (Bayes Room). If you need the Zoom link, please email stat.admin@austin.utexas.edu.
Share
Other Events in This Series
Apr
12
2024
Statistics Ph.D. Dissertation Defense - Shuying Wang
Bayesian Inference for Stochastic Compartmental Models and Marginal Cox Process
11:00 am – 1:00 pm • In Person
Speaker(s): Shuying Wang
Jul
26
2024
Statistics Ph.D. Dissertation Defense - Ciara Nugent
A Decision Theoretic Approach to Combining Inference Across Data Sources with Applications to Subgroup Analysis in Clinical Trials
8:30 am – 10:30 am • Virtual
Speaker(s): Ciara Nugent
Jul
29
2024
Statistics Ph.D. Dissertation Defense - Huangjie Zheng
Implicit Distributional Matching at High Dimensionality
11:00 am – 1:00 pm • Virtual
Speaker(s): Huangjie Zheng
Jul
31
2024
Statistics Ph.D. Dissertation Defense - Rimli Sengupta
Semi-Parametric Generalized Linear Models in Novel Analytical Contexts
9:45 am – 11:45 am • In Person
Speaker(s): Rimli Sengupta
Nov
15
2024
Statistics Ph.D. Dissertation Defense - Zhendong Wang
Enhancing Efficiency and Controllability in Generative Models for Reinforcement Learning and Robotics
8:00 am – 10:00 am • In Person
Speaker(s): Zhendong Wang
Apr
2
2025
Statistics Ph.D. Dissertation Defense - Michael Schwob
Bayesian Hierarchical Models for Dependent Ecological Data
1:00 pm – 3:00 pm • In Person
Apr
3
2025
Statistics Ph.D. Dissertation Defense - Rebecca Knowlton
Heterogeneous Surrogate Markers in Clinical Trials and Real-World Settings
2:00 pm – 4:00 pm • In Person
Apr
7
2025
Statistics Ph.D. Dissertation Defense - Angela Ting
Bayesian Nonparametric Methods for Heterogeneous Treatment and Mediation Effect Estimation
3:00 pm – 5:00 pm • In Person