Statistics Ph.D. Dissertation Defense - Huangjie Zheng
Jul
29
2024
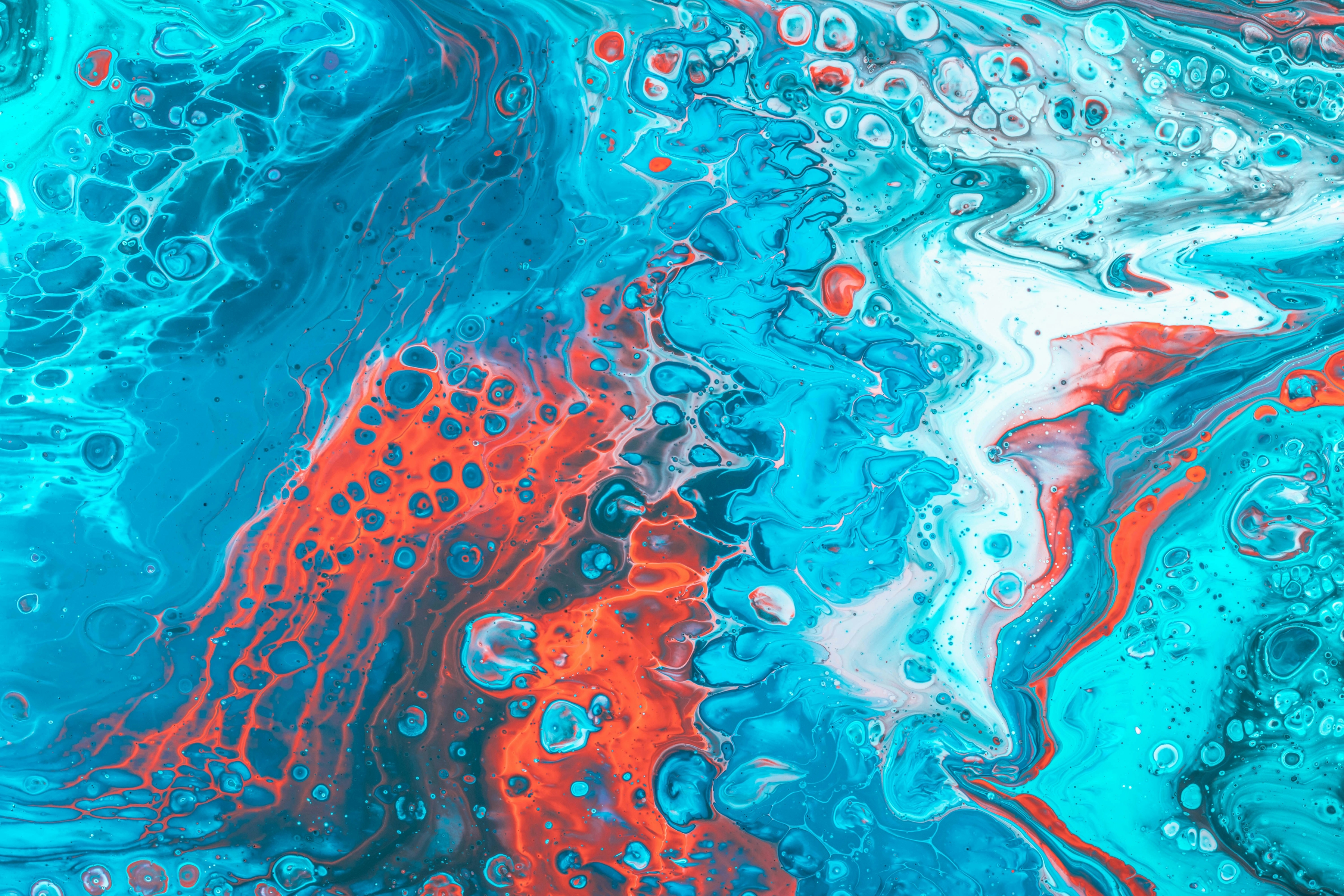
Jul
29
2024
Description
This 2024 Dissertation Defense will be held on Monday July 29, from 11:00 a.m. to 1:00 p.m. with Huangjie Zheng. This event will be virtual. If you need the Zoom link, please email stat.admin@austin.utexas.edu.
Title: Implicit Distributional Matching at High Dimensionality
Advisor: Mingyuan Zhou
Abstract: In the realm of high-dimensional data generative modeling, probabilistic models often provide robust and interpretable access to generate samples by estimating distributions. With the integration of deep neural networks, these probabilistic models become even more powerful in capturing complex data distributions. However, traditional statistical methods face significant challenges in efficiently building expressive distributions; for example, Maximum-Likelihood-Estimation (MLE) based methods tend to favor mode-covering, while reverse Kullback-Leibler (KL) based methods favor mode-seeking. These challenges limit the effectiveness of deep generative models in high-dimensional contexts. This dissertation pushes the boundary of implicit distributional matching (IDM) to address these challenges.
Implicit distributional matching leverages the capacity to estimate distributions using only samples, aligning data distributions without relying on explicit functional forms, thereby enabling flexible distribution modeling with deep neural networks in high-dimensional spaces. This research focuses on accurately estimating distributions and achieving a balance between mode-covering and mode-seeking. The dissertation is organized into three parts.
In the first part, we introduce novel implicit distributional matching methods that utilize both the chain rule and Bayes' theorem to address the dilemma of mode-covering versus mode-seeking. In the second part, we connect implicit distribution matching with diffusion-based (also known as score-matching-based) generative models, demonstrating how IDM complements the mode-seeking properties of diffusion models, thereby enhancing their efficiency and performance. In the final part, we showcase the application of IDM methods in achieving accurate and robust alignment of distributions across various data modalities and tasks, such as self-supervised pretraining and domain adaptation.
The findings of this dissertation illustrate that implicit distributional matching provides a flexible and powerful solution for distribution modeling in numerous machine learning problems, significantly improving the effectiveness of high-dimensional data generative models.
Location
Share
Other Events in This Series
Apr
12
2024
Statistics Ph.D. Dissertation Defense - Shuying Wang
Bayesian Inference for Stochastic Compartmental Models and Marginal Cox Process
11:00 am – 1:00 pm • In Person
Speaker(s): Shuying Wang
Jul
26
2024
Statistics Ph.D. Dissertation Defense - Ciara Nugent
A Decision Theoretic Approach to Combining Inference Across Data Sources with Applications to Subgroup Analysis in Clinical Trials
8:30 am – 10:30 am • Virtual
Speaker(s): Ciara Nugent
Jul
31
2024
Statistics Ph.D. Dissertation Defense - Rimli Sengupta
Semi-Parametric Generalized Linear Models in Novel Analytical Contexts
9:45 am – 11:45 am • In Person
Speaker(s): Rimli Sengupta
Nov
15
2024
Statistics Ph.D. Dissertation Defense - Zhendong Wang
Enhancing Efficiency and Controllability in Generative Models for Reinforcement Learning and Robotics
8:00 am – 10:00 am • In Person
Speaker(s): Zhendong Wang
Apr
2
2025
Statistics Ph.D. Dissertation Defense - Michael Schwob
Bayesian Hierarchical Models for Dependent Ecological Data
1:00 pm – 3:00 pm • In Person
Apr
3
2025
Statistics Ph.D. Dissertation Defense - Rebecca Knowlton
Heterogeneous Surrogate Markers in Clinical Trials and Real-World Settings
2:00 pm – 4:00 pm • In Person
Apr
7
2025
Statistics Ph.D. Dissertation Defense - Angela Ting
Bayesian Nonparametric Methods for Heterogeneous Treatment and Mediation Effect Estimation
3:00 pm – 5:00 pm • In Person
Apr
11
2025
Statistics Ph.D. Dissertation Defense - Fuheng Cui
Bayesian Predictive Posterior Distributions
1:00 pm – 3:00 pm • In Person