Statistics Ph.D. Dissertation Defense - Michael Schwob
Apr
2
2025
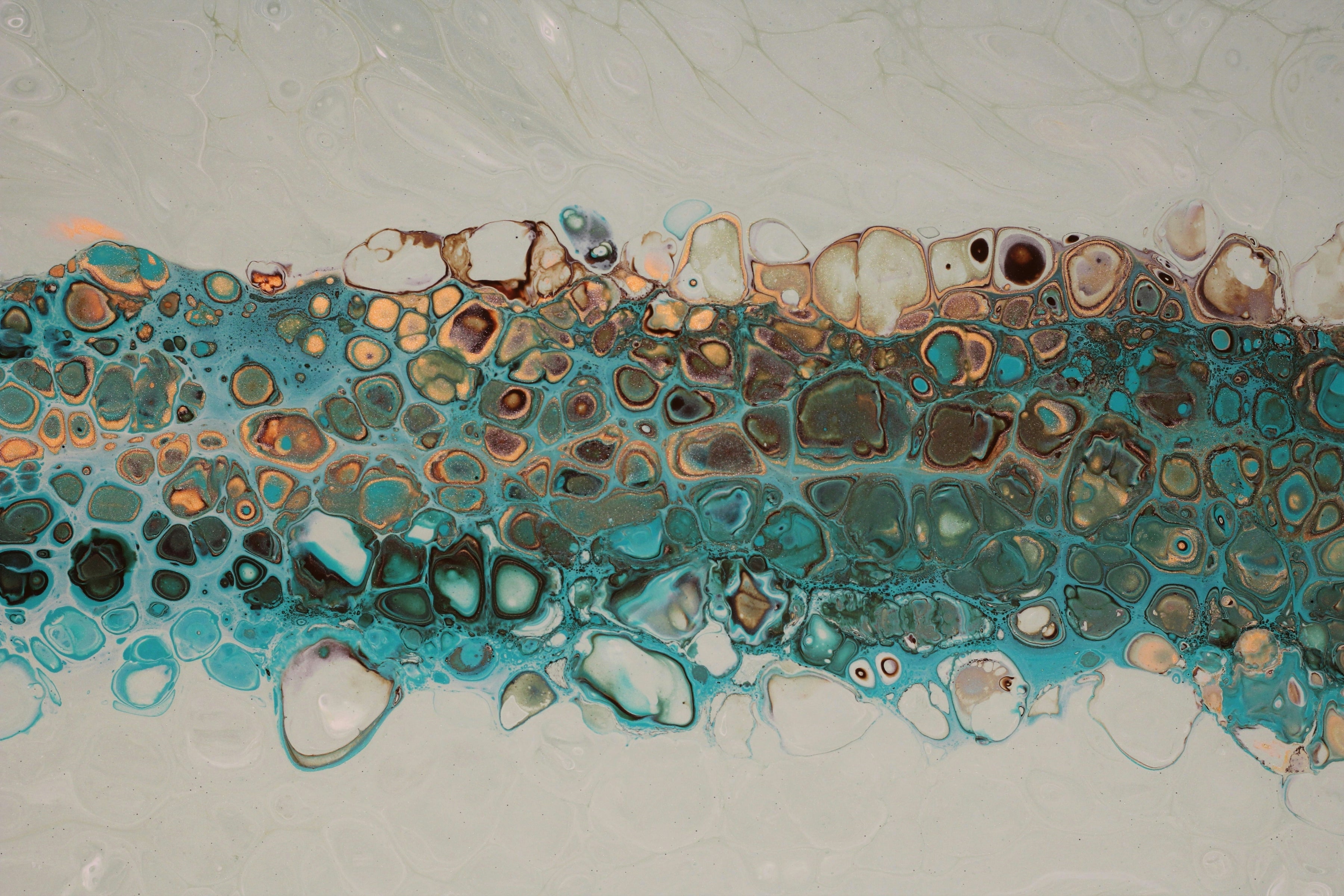
Apr
2
2025
Description
This 2025 Dissertation Defense will be held on Wednesday, April 2 from 1:00 p.m. to 3:00 p.m. with Michael Schwob. This event will be hybrid. If you are able to attend in person, it will be held in WEL 5.204. If you need the Zoom link, please email stat.admin@austin.utexas.edu.
Title: Bayesian Hierarchical Models for Dependent Ecological Data
Advisor: Dr. Mevin Hooten
Abstract: Ecological data are inherently dependent due to spatial and temporal autocorrelation, species interactions, seasonality, and environmental gradients. In this dissertation, I developed statistical methods that account for various forms of dependence that arise in ecological data. I first proposed a dynamic population model for the analysis of abundance data, which often experience a high degree of temporal autocorrelation. The model was fit to mosquito abundance data collected across North America. An additional source of dependence came in the form of temporal preferential sampling (TPS), where data collection was informed by the observed abundance. I accounted for temporal autocorrelation and TPS in a Bayesian hierarchical model (BHM), which was specified mechanistically such that the observed abundance was related to the Gompertz growth function. Due to the mechanistic specification, inference was made for abundance and phenological quantities of interest. Then, I developed a BHM to infer the relationship between population genetic data and the environment. Such data arise in the field of landscape genomics, where there is interest in the functional connectivity and migration patterns of populations. Population genetic data are highly dependent, and I accounted for spatial and temporal autocorrelation via composite likelihoods. The BHM contains a dyadic regression, which is linked to an advection-diffusion differential equation to infer how Bronze Age humans migrated throughout Europe. Finally, I developed a BHM for the analysis of spatial compositional data, which often arise in community ecology, organismal composition studies, and ecosystem forecasting. The model accounts for spatial autocorrelation and the compositional nature of the data. In particular, I transformed the compositional data to directional data and modeled it using a novel hyperspheric distribution. This is the first spatial hyperspheric approach to contain fixed and latent spatial random effects, while accounting for the compositional constraints inherent to the data. I applied the model to bioacoustic signal classifications obtained from a machine learning classifier.
Location
This event will be hybrid. If you are able to attend in person, it will be held in WEL 5.204. If you need the Zoom link, please email stat.admin@austin.utexas.edu.
Share
Other Events in This Series
Apr
12
2024
Statistics Ph.D. Dissertation Defense - Shuying Wang
Bayesian Inference for Stochastic Compartmental Models and Marginal Cox Process
11:00 am – 1:00 pm • In Person
Speaker(s): Shuying Wang
Jul
26
2024
Statistics Ph.D. Dissertation Defense - Ciara Nugent
A Decision Theoretic Approach to Combining Inference Across Data Sources with Applications to Subgroup Analysis in Clinical Trials
8:30 am – 10:30 am • Virtual
Speaker(s): Ciara Nugent
Jul
29
2024
Statistics Ph.D. Dissertation Defense - Huangjie Zheng
Implicit Distributional Matching at High Dimensionality
11:00 am – 1:00 pm • Virtual
Speaker(s): Huangjie Zheng
Jul
31
2024
Statistics Ph.D. Dissertation Defense - Rimli Sengupta
Semi-Parametric Generalized Linear Models in Novel Analytical Contexts
9:45 am – 11:45 am • In Person
Speaker(s): Rimli Sengupta
Nov
15
2024
Statistics Ph.D. Dissertation Defense - Zhendong Wang
Enhancing Efficiency and Controllability in Generative Models for Reinforcement Learning and Robotics
8:00 am – 10:00 am • In Person
Speaker(s): Zhendong Wang
Apr
3
2025
Statistics Ph.D. Dissertation Defense - Rebecca Knowlton
Heterogeneous Surrogate Markers in Clinical Trials and Real-World Settings
2:00 pm – 4:00 pm • In Person
Apr
7
2025
Statistics Ph.D. Dissertation Defense - Angela Ting
Bayesian Nonparametric Methods for Heterogeneous Treatment and Mediation Effect Estimation
3:00 pm – 5:00 pm • In Person
Apr
11
2025
Statistics Ph.D. Dissertation Defense - Fuheng Cui
Bayesian Predictive Posterior Distributions
1:00 pm – 3:00 pm • In Person