SDS Seminar Series – Dr. Bodhisattva Sen
Apr
26
2024
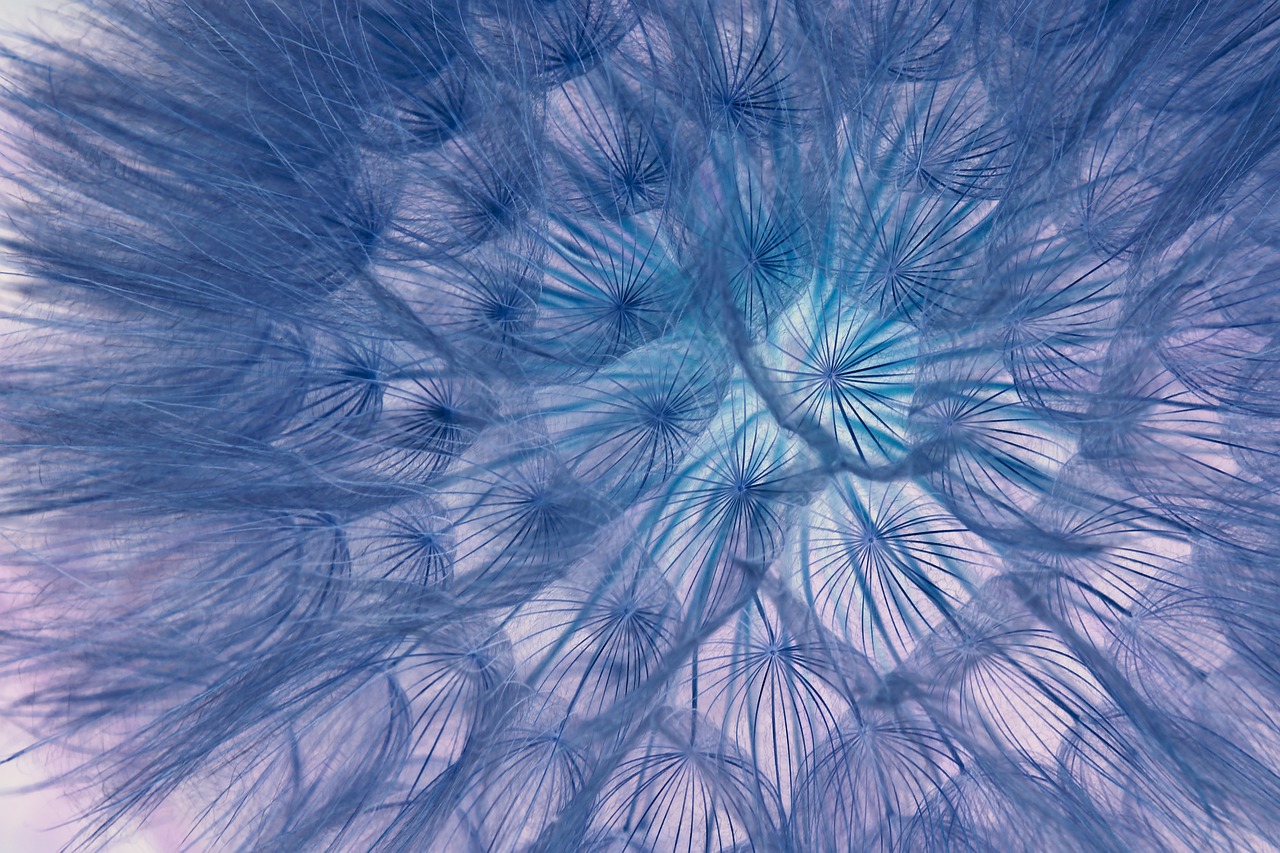
Apr
26
2024
Description
The Spring 2024 SDS Seminar Series continues on April 26th from 2:00 p.m. to 3:00 p.m. with Dr. Bodhisattva Sen (Statistics, Columbia University). This event is in-person.
Title: Extending the Scope of Nonparametric Empirical Bayes
Abstract: In this talk we will describe two applications of empirical Bayes (EB) methodology. EB procedures estimate the prior probability distribution in a latent variable model or Bayesian model from the data. In the first part we study the (Gaussian) signal plus noise model with multivariate, heteroscedastic errors. This model arises in many large-scale denoising problems (e.g., in astronomy). We consider the nonparametric maximum likelihood estimator (NPMLE) in this setting. We study the characterization, uniqueness, and computation of the NPMLE which estimates the unknown (arbitrary) prior by solving an infinite-dimensional convex optimization problem. The EB posterior means based on the NPMLE have low regret, meaning they closely target the oracle posterior means one would compute with the true prior in hand. We demonstrate the adaptive and near-optimal properties of the NPMLE for density estimation, denoising and deconvolution.
In the second half of the talk, we consider the problem of Bayesian high dimensional regression where the regression coefficients are drawn i.i.d. from an unknown prior. To estimate this prior distribution, we propose and study a "variational empirical Bayes" approach — it combines EB inference with a variational approximation (VA). The idea is to approximate the intractable marginal log-likelihood of the response vector --- also known as the "evidence" --- by the evidence lower bound (ELBO) obtained from a naive mean field (NMF) approximation. We then maximize this lower bound over a suitable class of prior distributions in a computationally feasible way. We show that the marginal log-likelihood function can be (uniformly) approximated by its mean field counterpart. More importantly, under suitable conditions, we establish that this strategy leads to consistent approximation of the true posterior and provides asymptotically valid posterior inference for the regression coefficients.
Location
Peter O’Donnell Jr. Building (POB) 2.302
Share
Other Events in This Series
Sep
8
2023
SDS Seminar Series – Dr. Emily Roberts
A Causal Inference Approach for Surrogate Marker Evaluation with Mixed Models
2:00 pm – 3:00 pm • In Person
Speaker(s): Emily Roberts
Sep
15
2023
SDS Seminar Series – Dr. Dimitris Korobilis
Monitoring Multicountry Macroeconomic Risk
2:00 pm – 3:00 pm • Virtual
Speaker(s): Dimitris Korobilis
Sep
22
2023
SDS Seminar Series – Dr. Will Fithian
Estimating the False Discovery Rate of Model Selection
2:00 pm – 3:00 pm • In Person
Speaker(s): Will Fithian
Sep
29
2023
SDS Seminar Series – Dr. David Moriarty
A Data Science Journey in Business
2:00 pm – 3:00 pm • In Person
Speaker(s): David Moriarty
Oct
6
2023
SDS Seminar Series – Dr. Amanda Ellis
Navigating the Future of Statistics Education: Leveraging ChatGPT's Advantages and Overcoming Challenges
2:00 pm – 3:00 pm • Virtual
Speaker(s): Amanda Ellis
Oct
20
2023
SDS Seminar Series – Dr. Amy Zhang
Bisimulation and Reinforcement Learning
2:00 pm – 3:00 pm • Virtual
Speaker(s): Amy Zhang
Oct
27
2023
SDS Seminar Series – Dr. Marcelo Medeiros
Global Inflation Forecasting: Benefits from Machine Learning Methods
2:00 pm – 3:00 pm • Virtual
Speaker(s): Marcelo Medeiros
Nov
3
2023
SDS Seminar Series - Dr. Steve Yadlowsky
Choosing a Proxy Metric from Past Experiments
2:00 pm – 3:00 pm • Virtual
Speaker(s): Steve Yadlowsky
Nov
10
2023
SDS Seminar Series – Drew Herren
Statistical Aspects of SHAP: Functional ANOVA for Model Interpretation
2:00 pm – 3:00 pm • In Person
Speaker(s): Drew Herren
Dec
1
2023
SDS Seminar Series – Dr. Dave Zhao
High-Dimensional Nonparametric Empirical Bayes Problems in Genomics
2:00 pm – 3:00 pm • In Person
Speaker(s): Dave Zhao