SDS Seminar Series – Dr. Bodhisattva Sen
Apr
26
2024
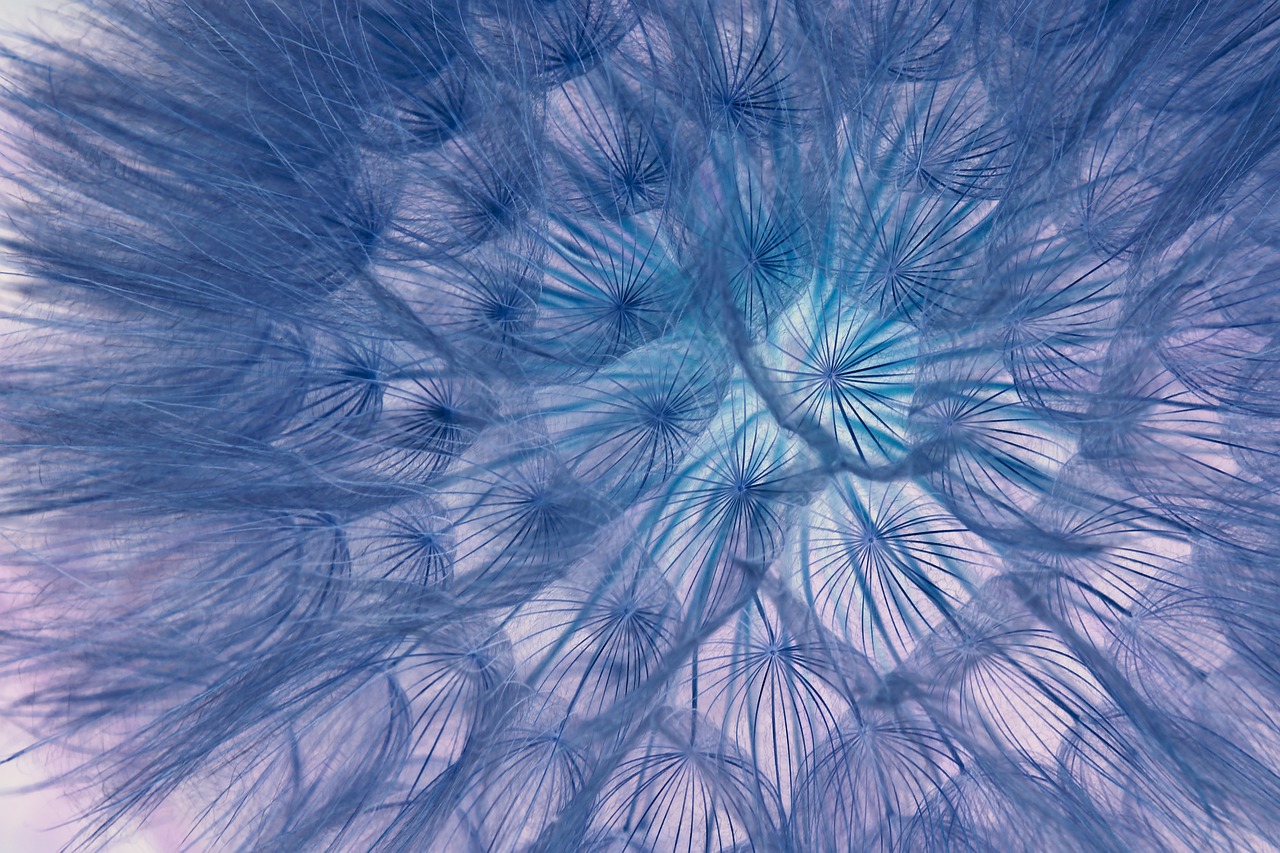
Apr
26
2024
Description
The Spring 2024 SDS Seminar Series continues on April 26th from 2:00 p.m. to 3:00 p.m. with Dr. Bodhisattva Sen (Statistics, Columbia University). This event is in-person.
Title: Extending the Scope of Nonparametric Empirical Bayes
Abstract: In this talk we will describe two applications of empirical Bayes (EB) methodology. EB procedures estimate the prior probability distribution in a latent variable model or Bayesian model from the data. In the first part we study the (Gaussian) signal plus noise model with multivariate, heteroscedastic errors. This model arises in many large-scale denoising problems (e.g., in astronomy). We consider the nonparametric maximum likelihood estimator (NPMLE) in this setting. We study the characterization, uniqueness, and computation of the NPMLE which estimates the unknown (arbitrary) prior by solving an infinite-dimensional convex optimization problem. The EB posterior means based on the NPMLE have low regret, meaning they closely target the oracle posterior means one would compute with the true prior in hand. We demonstrate the adaptive and near-optimal properties of the NPMLE for density estimation, denoising and deconvolution.
In the second half of the talk, we consider the problem of Bayesian high dimensional regression where the regression coefficients are drawn i.i.d. from an unknown prior. To estimate this prior distribution, we propose and study a "variational empirical Bayes" approach — it combines EB inference with a variational approximation (VA). The idea is to approximate the intractable marginal log-likelihood of the response vector --- also known as the "evidence" --- by the evidence lower bound (ELBO) obtained from a naive mean field (NMF) approximation. We then maximize this lower bound over a suitable class of prior distributions in a computationally feasible way. We show that the marginal log-likelihood function can be (uniformly) approximated by its mean field counterpart. More importantly, under suitable conditions, we establish that this strategy leads to consistent approximation of the true posterior and provides asymptotically valid posterior inference for the regression coefficients.
Location
Peter O’Donnell Jr. Building (POB) 2.302
Share
Other Events in This Series
Mar
1
2024
SDS Seminar Series – Dr. Laura Hatfield
Predict, Correct, Select: A New General Identification Strategy for Controlled Pre-Post Designs
2:00 pm – 3:00 pm • Virtual
Speaker(s): Laura Hatfield
Mar
22
2024
SDS Seminar Series – Dr. Sivaraman Balakrishnan
Statistical Inference for Optimal Transport
2:00 pm – 3:00 pm • In Person
Speaker(s): Sivaraman Balakrishnan
Mar
29
2024
SDS Seminar Series – Dr. Purna Sarkar
Some New Results for Streaming Principal Component Analysis
2:00 pm – 3:00 pm • In Person
Speaker(s): Purna Sarkar
Apr
12
2024
SDS Seminar Series – Dr. Daniela Witten
Data Thinning and Its Applications
2:00 pm – 3:00 pm • In Person
Apr
19
2024
SDS Seminar Series – Dr. William Rosenberger
Design and Inference for Enrichment Trials with a Continuous Biomarker
2:00 pm – 3:00 pm • In Person
Speaker(s): William Rosenberger
Sep
6
2024
SDS Seminar Series – Christine Peterson, University of Texas MD Anderson Cancer Center
New Methods for Microbiome Data Integration
2:00 pm – 3:00 pm • In Person
Speaker(s): Christine Peterson
Sep
13
2024
SDS Seminar Series – Matthew Vanaman, University of Texas at Austin
Data Analysis from the Zoo to the Wild and Back
2:00 pm – 3:00 pm • In Person
Speaker(s): Matthew Vanaman
Sep
20
2024
SDS Seminar Series – Saptarshi Roy, University of Texas at Austin
On the Computational Complexity of Private High-dimensional Model Selection
2:00 pm – 3:00 pm • In Person
Speaker(s): Saptarshi Roy
Sep
27
2024
SDS Seminar Series – Abhra Sarkar, University of Texas at Austin
(Bayesian) Semiparametric Local Inference (and Other Stories)
2:00 pm – 3:00 pm • In Person
Speaker(s): Abhra Sarkar
Oct
4
2024
SDS Seminar Series – Huiyan Sang, Texas A&M University
GS-BART: Graph Split Additive Decision Trees for Spatial and Network Data
2:00 pm – 3:00 pm • In Person
Speaker(s): Huiyan Sang